Inductive Bias Transfer
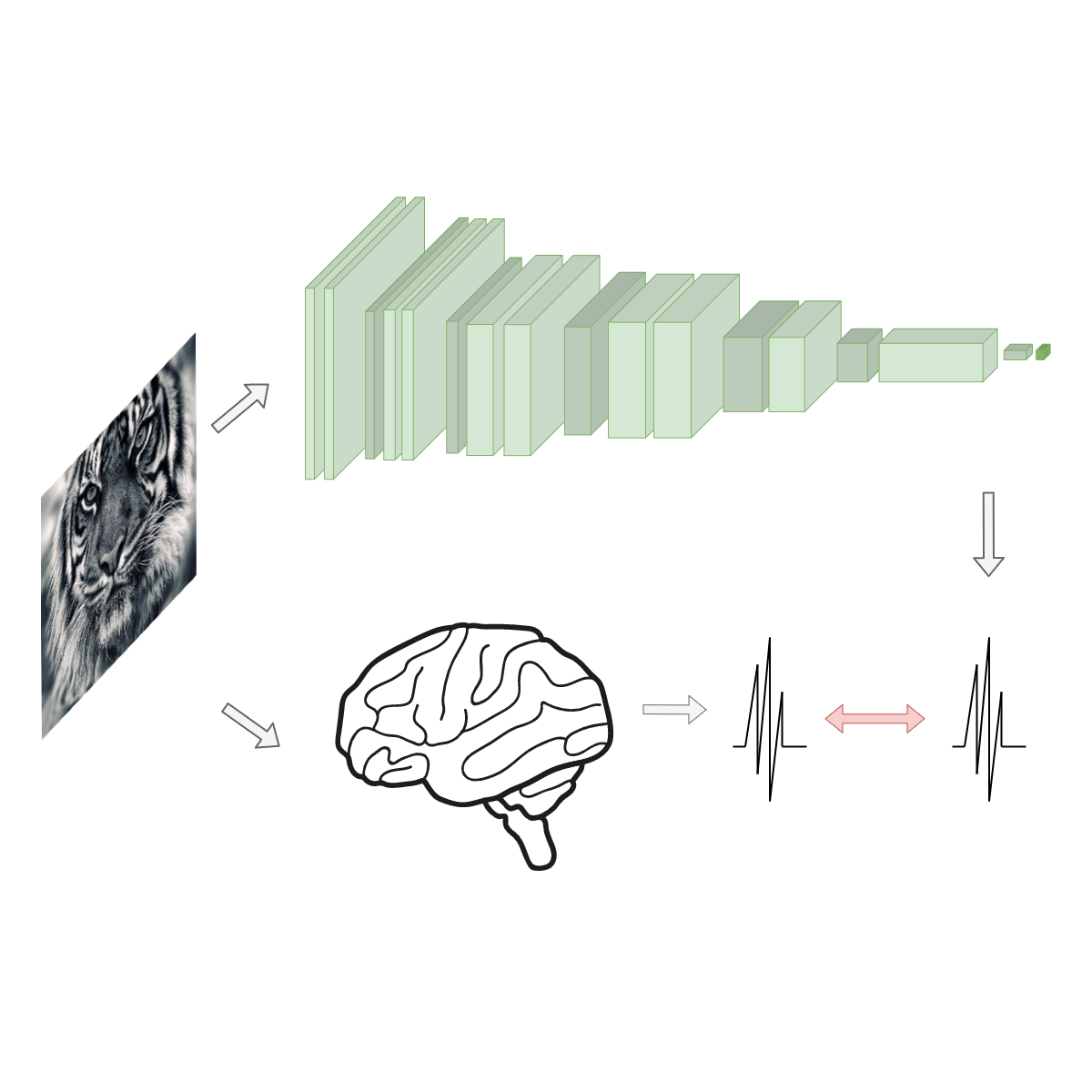
The mammalian visual system generalizes robustly across a wide range of perturbations and changes of contexts, while state of the art computer vision networks are easily thrown off. One way to explain this generalization ability are better inductive biases encoded in the representations of visual stimuli throughout the visual cortex. We are studying mechanisms to transfer inductive biases between brains and machines to improve the generalization ability of artificial networks, and get a better understanding of what lets real brains generalize so well.
Lab members are shown in this color.
2023
Arne Nix, Max Burg, Fabian Sinz
HARD: Hard Augmentations for Robust Distillation
arXiv
|
2022
Arne Nix, Max Burg, Fabian Sinz
Leading by example: Guiding knowledge transfer with adversarial data augmentation
Neurips 2022 Workshop SyntheticData4ML
|
Arne Nix, Suhas Shrinivasan, Edgar Y. Walker, Fabian H. Sinz
Can Functional Transfer Methods Capture Simple Inductive Biases?
AISTATS 2022
|
2021
Shahd Safarani, Arne Nix, Konstantin Willeke, Santiago A. Cadena, Kelli Restivo, George Denfield, Andreas S. Tolias, Fabian H. Sinz
Towards robust vision by multi-task learning on monkey visual cortex
NeurIPS
|
Shahd Safarani, Arne Nix, Konstantin Willeke, Santiago A. Cadena, Kelli Restivo, George Denfield, Andreas S. Tolias, Fabian H. Sinz
Towards robust vision by multi-task learning on monkey visual cortex
ICLR 2021 Workshop: How Can Findings About The Brain Improve AI Systems?
|
2019
Zhe Li, Wieland Brendel, Edgar Walker, Erick Cobos, Taliah Muhammad, Jacob Reimer, Matthias Bethge, Fabian Sinz, Xaq Pitkow, Andreas Tolias
Learning from brains how to regularize machines
NeurIPS (accepted)
|
Fabian H. Sinz, Xaq Pitkow, Jacob Reimer, Matthias Bethge, Andreas S. Tolias
Engineering a less artificial intelligence
Neuron 103(6)
|
2017
M. Ren, R. Liao, R. Urtasun, F. H. Sinz, R. S. Zemel
Normalizing the normalizers: Comparing and extending network normalization schemes
ICLR
|
T. Nguyen, W. Lui, F. Sinz, R. G. Araniuk, A. S. Tolias, X. Pitkow, A. B. Patel
Towards a Cortically Inspired Deep Learning Model: Semi-Supervised Learning, Divisive Normalization, and Synaptic Pruning
CCN
|