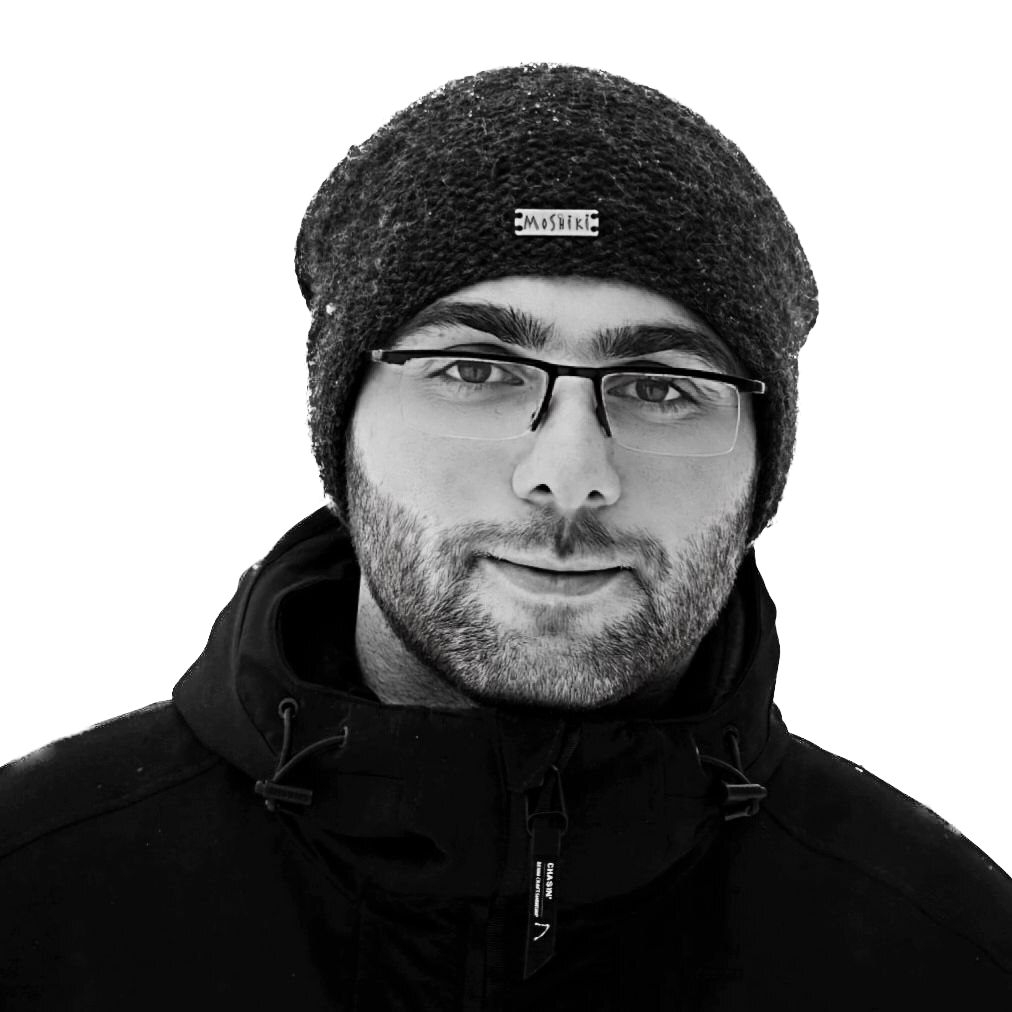
My interest lies in identifying regularities underlying neural computations that contribute to data-efficient learning and robust inference. These regularities are commonly framed as tuning where the activity of many neurons is sensitive to a common axis (aka tuning axis) in the input space. For instance, in the context of the visual system, many neurons have similar receptive fields but each is rotated differently, which makes the rotation angle the tuning axis. The questions I am currently working on are: 1) What other regularities are there in the computations performed by cortical neuronal populations? 2) Can we develop methods that automatically discover tuning in a given multiple-input and multiple-output (MIMO) system? And 3) How can we use models to design experiments such that we can control the activity of neuronal populations and ultimately control learning and inference. To answer these questions I use deep neural networks as models of the visual system and work with large scale neuro-physiological and -anatomical data.
Lab members are shown in this color.
2025
Mohammad Bashiri1, Luca Baroni1, Jan Antolik, Fabian H. Sinz
Learning and aligning single-neuron invariance manifolds in visual cortex
ICLR (oral; <1.8% submissions; 34. overall; 1. neuroscience)
, equal contribution: 1
|
2024
Polina Turishcheva, Paul G. Fahey, Michaela Vystrčilová, Laura Hansel, Rachel E Froebe, Kayla Ponder, Yongrong Qiu, Konstantin Friedrich Willeke, Mohammad Bashiri, Ruslan Baikulov, Yu Zhu, Lei Ma, Shan Yu, Tiejun Huang, Bryan M. Li, Wolf De Wulf, Nina Kudryashova, Matthias H. Hennig, Nathalie Rochefort, Arno Onken, Eric Wang, Zhiwei Ding, Andreas S. Tolias, Fabian H. Sinz, Alexander S Ecker
Retrospective for the Dynamic Sensorium Competition for predicting large-scale mouse primary visual cortex activity from videos
NeurIPS 2024
|
Jiakun Fu, Pawel A. Pierzchlewicz, Konstantin F. Willeke, Mohammad Bashiri, Taliah Muhammad, Maria Diamantaki, Emmanouil Froudarakis, Kelli Restivo, Kayla Ponder, George H. Denfield, Fabian H. Sinz, Andreas S. Tolias, Katrin Franke
Heterogeneous orientation tuning in primary visual cortex of mice diverges from Gabor-like receptive fields in primates
Cell Reports
|
Mohammad Bashiri
PhD Thesis, International Max Planck Research School for Intelligent Systems, Tübingen
|
2023
Konstantin F. Willeke, Paul G. Fahey, Mohammad Bashiri, Laura Hansel, Christoph Blessing, Konstantin-Klemens Lurz, Max F. Burg, Santiago A. Cadena, Zhiwei Ding, Kayla Ponder, Taliah Muhammad, Saumil S. Patel, Kaiwen Deng, Yuanfang Guan, Yiqin Zhu, Kaiwen Xiao, Xiao Han, Simone Azeglio, Ulisse Ferrari, Peter Neri, Olivier Marre, Adrian Hoffmann, Kirill Fedyanin, Kirill Vishniakov, Maxim Panov, Subash Prakash, Kishan Naik, Kantharaju Narayanappa, Alexander S. Ecker, Andreas S. Tolias, Fabian H. Sinz
Retrospective on the SENSORIUM 2022 competition
Proceedings of Machine Learning Research
|
Polina Turishcheva, Paul G. Fahey, Laura Hansel, Rachel Froebe, Kayla Ponder, Michaela Vystrčilová, Konstantin F. Willeke, Mohammad Bashiri, Eric Wang, Zhiwei Ding, Andreas S. Tolias, Fabian H. Sinz, Alexander S. Ecker
The Dynamic Sensorium competition for predicting large-scale mouse visual cortex activity from videos
arXiv
|
Paweł A. Pierzchlewicz, Mohammad Bashiri, R. James Cotton, Fabian H. Sinz
Optimizing MPJPE promotes miscalibration in multi-hypothesis human pose lifting
ICLR - tiny paper
|
Konstantin-Klemens Lurz1, Mohammad Bashiri1, Edgar Y. Walker, Fabian H. Sinz
Bayesian Oracle for bounding information gain in neural encoding models
ICLR 2023
, equal contribution: 1
|
2022
Paweł A. Pierzchlewicz, R. James Cotton, Mohammad Bashiri, Fabian H. Sinz
Multi-hypothesis 3D human pose estimation metrics favor miscalibrated distributions
arXiv
|
Konstantin Lurz, Mohammad Bashiri, Fabian Sinz
Bayesian Oracle for bounding information gain in neural encoding models
Neurips 2022 Workshop InfoCog
|
Luca Baroni1, Mohammad Bashiri1, Konstantin Friedrich Willeke, Ján Antolík, Fabian Sinz
Learning Invariance Manifolds of Visual Sensory Neurons
Neurips 2022 Workshop NeurReps
, equal contribution: 1
|
Konstantin F. Willeke, Paul G. Fahey, Mohammad Bashiri, Laura Pede, Max F. Burg, Christoph Blessing, Santiago A. Cadena, Zhiwei Ding, Konstantin-Klemens Lurz, Kayla Ponder, Taliah Muhammad, Saumil S. Patel, Alexander S. Ecker, Andreas S. Tolias, Fabian H. Sinz
The Sensorium competition on predicting large-scale mouse primary visual cortex activity
arXiv
|
2021
Mohammad Bashiri, Edgar Y. Walker, Konstantin-Klemens Lurz, Akshay Kumar Jagadish, Taliah Muhammad, Zhiwei Ding, Zhuokun Ding, Andreas S. Tolias, Fabian H. Sinz
A flow-based latent state generative model of neural population responses to natural images
NeurIPS (spotlight)
conference paper
·
system identification
·
openreview
twitter
teaser-youtube
talk-youtube
github
simulation demo
biorXiv
|
Konstantin-Klemens Lurz, Mohammad Bashiri, Konstantin Friedrich Willeke, Akshay Kumar Jagadish, Eric Wang, Edgar Y Walker, Santiago Cadena, Taliah Muhammad, Eric Cobos, Andreas Tolias, Alexander Ecker, Fabian Sinz
Generalization in data-driven models of primary visual cortex
ICLR (spotlight)
|