Inception Loops
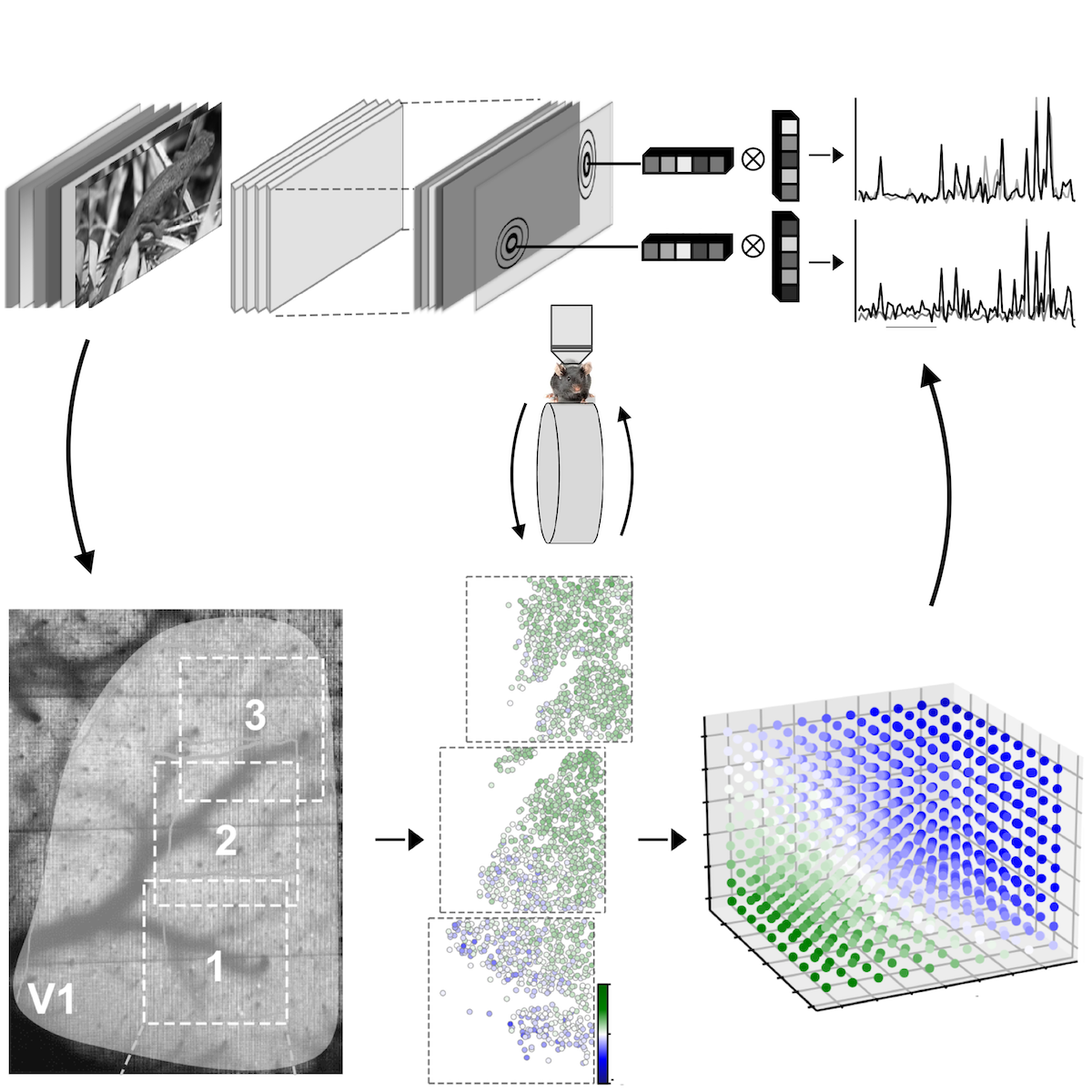
We are using highly predictive deep learning models as in silico avatars of neural populations in visual cortex and analyse their nonlinear properties. Our goal is to find interpretable and experimentally verifiable computational principles of biological vision. We do this by synthesizing new stimuli for neuroscientific experiments in silico and verifying them in vivo with our collaboration partners. We call this cycle “Inception Loops”.
Lab members are shown in this color.
2024
Jiakun Fu, Pawel A. Pierzchlewicz, Konstantin F. Willeke, Mohammad Bashiri, Taliah Muhammad, Maria Diamantaki, Emmanouil Froudarakis, Kelli Restivo, Kayla Ponder, George H. Denfield, Fabian H. Sinz, Andreas S. Tolias, Katrin Franke
Heterogeneous orientation tuning in primary visual cortex of mice diverges from Gabor-like receptive fields in primates
Cell Reports
|
2022
Erick Cobos, Taliah Muhammad, Paul G Fahey, Zhiwei Ding, Zhuokun Ding, Jacob Reimer, Fabian H. Sinz, Andreas Tolias
It takes neurons to understand neurons: Digital twins of visual cortex synthesize neural metamers
biorXiv
|
Katrin Franke1, Konstantin F. Willeke1, Kayla Ponder, Mario Galdamez, Taliah Muhammad, Saumil Patel, Emmanouil Froudarakis, Jacob Reimer, Fabian H. Sinz, Andreas S. Tolias
State-dependent pupil dilation rapidly shifts visual feature selectivity
Nature
, equal contribution: 1
|
2019
Edgar Y. Walker1, Fabian H. Sinz1, Erick Cobos, Taliah Muhammad, Emmanouil Froudarakis, Paul G. Fahey, Alexander S. Ecker, Jacob Reimer, Xaq Pitkow, Andreas S. Tolias
Inception loops discover what excites neurons most using deep predictive models
Nature Neuroscience
, equal contribution: 1
|